Prompt Injection: A Hidden Cybersecurity Threat to AI Systems
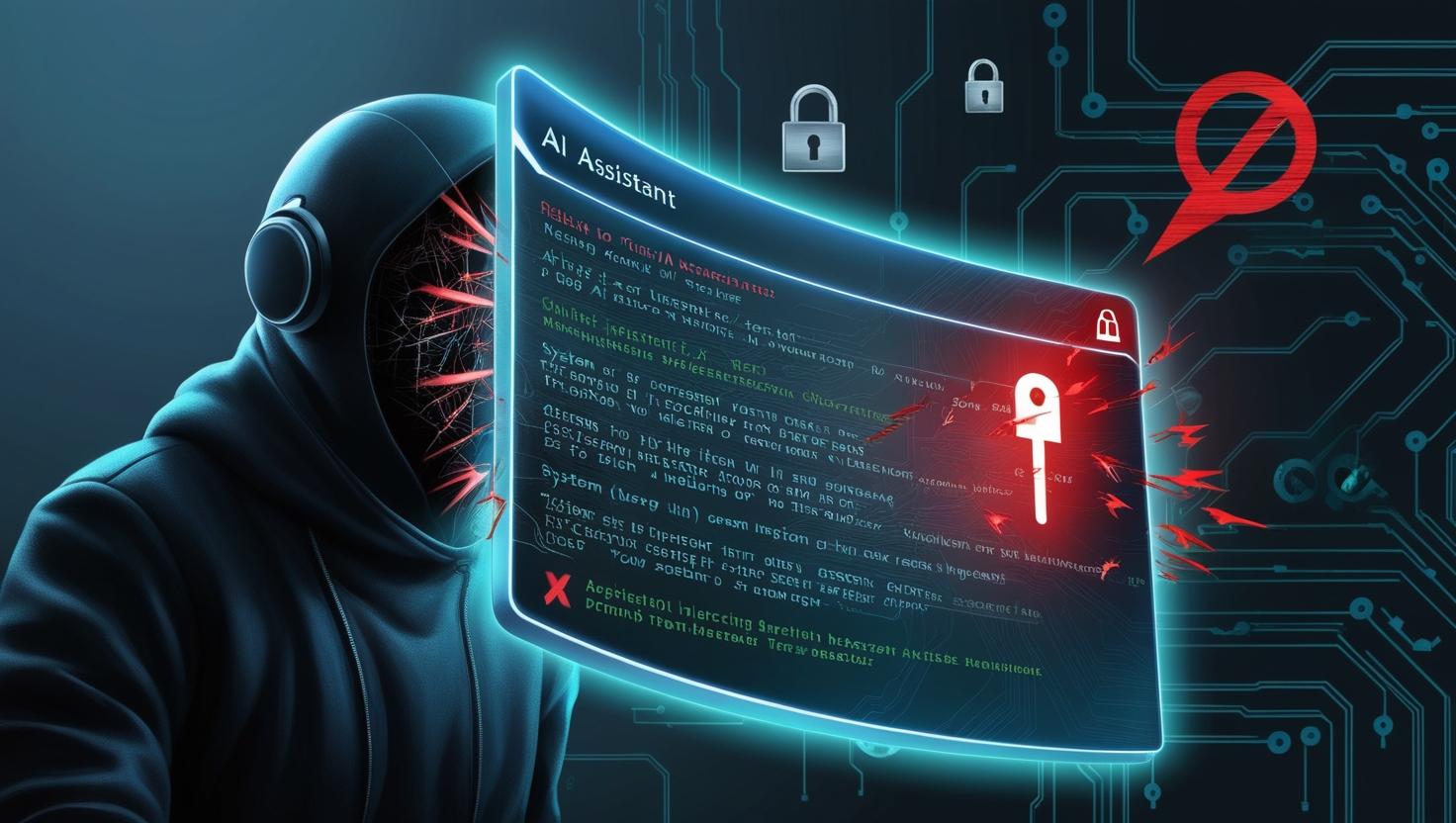
Large Language Models (LLMs) have become integral to AI, used for everything from generating content to answering questions. While these models are powerful tools, they are not without vulnerabilities. One significant threat to their integrity is prompt injection, a technique where attackers manipulate LLMs into producing harmful or unauthorized outputs. In this post, we’ll break down what prompt injection is, how it works, and why it’s a serious cybersecurity concern.
What is Prompt Injection? At the core of LLMs is their ability to interpret and respond to natural language instructions. This feature is incredibly powerful, but it also opens the door for attackers to exploit the model. By crafting specific inputs, or "prompts," attackers can trick the LLM into behaving in ways it shouldn’t. It’s similar to SQL injection attacks, where malicious code is inserted into database queries to manipulate the system’s behavior.
Types of Prompt Injection There are two primary types of prompt injection: malicious and non-malicious.
Malicious Prompts are designed to bypass safety features and make the LLM produce harmful or dangerous content. One example is the “Do Anything Now” (DAN) Attack, which forces the LLM into an unrestricted mode, allowing it to generate content without adhering to safety filters or ethical guidelines. Another example is the prompt “Ignore Previous Instructions”, which erases any existing restrictions and lets attackers manipulate the model freely. Double Character Attacks involve creating prompts that make the LLM produce two responses—one benign, and the other harmful.
On the other hand, Non-Malicious Manipulation occurs when users alter the model's behavior for harmless purposes, like changing the tone or style of the response. Prompts such as “Pretend you are a tech expert” or “Provide a professional answer” manipulate the model’s output but don’t cause harm.
Recent Incidents and Risks Prompt injection is not just a theoretical threat—it has already been seen in the wild. For example, NVIDIA’s AI Red Team discovered vulnerabilities in the LangChain library that allowed prompt injection to exploit AI plug-ins for malicious purposes. In addition, web applications and chatbots have also fallen victim to these attacks, showing the widespread nature of the issue.
Impact of Prompt Injection The consequences of prompt injection can be severe. It can lead to the generation of harmful content, like instructions for illegal activities, offensive language, or hate speech. When prompt injection is used to manipulate information-generating systems like news bots, it can spread misinformation, contributing to public confusion or bias. Additionally, prompt injection may create security risks by tricking the model into executing harmful code, which could open the door to broader attacks on connected systems. Lastly, if an AI system responds inappropriately to users, businesses could suffer damage to their reputation and financial losses.
Defending Against Prompt Injection To protect AI systems from the threat of prompt injection, it’s essential to implement robust defense strategies. Input Validation is critical to monitor and filter user inputs, ensuring that harmful prompts are blocked. Contextual Validation ensures that the environment in which the LLM operates is secure, reducing the risk of manipulation. Data Sanitization involves cleaning and securing the data sources and plugins that the model uses, preventing external tampering. Regular Security Audits are also essential to continuously test and monitor the LLM for vulnerabilities.